There are a lot of COVID-19 tests
which quote figures on sensitivity and accuracy, all very high, and if you read the fine print they will define precisely conditions such as “seven days after onset of symptoms”. But what does this all mean especially when the general public (which is you and me whether you are a scientist or not) want a test that tells them if they have the disease unknowingly “when they don’t have symptoms”?
Let us start with sensitivity. Quite straight forward: If I have 100 patients with COVID-19 how many score positive in the test? Ninety-five of the 100; then you have a sensitivity of 95%, Excellent! So we have 95 true positives and 5 false negatives. But this is when things start to get slightly tricky.
At what stage of the disease did you do the test, full-blown hospitalisation? 7 days after symptoms began? 1st day of symptoms? Before symptoms began?!
Ok, let us look at the other side of the coin. If I have 100 people without COVID-19 (I am ignoring that they might have a different Coronavirus and assuming perfectly healthy individuals), how many score positive? 1 in a 100, so that is 1% false positive but 99% true negative. That test has the specificity of 99%.
From all these numbers we can calculate the accuracy of a test.
True positives + True negatives
True positives + True negatives + False positives +False negatives
So that for our hypothetical test this is 95+99/95+99+5+1 = 97%
This all sounds wonderful but here comes the issue of positive predictive value and what it means if you score positive on a test for COVID-19 and you have no symptoms.
What is the probability you are actually infected under these circumstances?
Well, first thing we have to realise is that in the accuracy calculation above we assumed that we have an equal number of infected people as we do non-infected. Well we know that is not true, so let us assume that 1 in 3000 people are infected and spreading the virus.
- We keep the same sensitivity and specificity rates, but for every 1 true positives 31 true negative will be scored as positive (still 1% of the true negative population).
- Thus the probability of the test meaning you have the disease if you don’t have symptoms equals true positive number/true positive and false positive = 1/32 = about 30% (positive predictive value).
Same sensitivity and specificity, same accuracy but the lower the incidence of disease (COVID-19 infected individuals) mean you pick up a lot of false positives.
- If the incidence of COVID infection in the population is 1 in 100,000 then the positive predictive value is only 0.1% chance of being correct.
For a test that is only 70% accurate your positive predictive value could fall very low indeed.
So what is the point of these tests?
Well if you have symptoms then testing is very sensible; and, for governments, testing randomly is important to get an estimate of the incidence and spread. As the incidence of COVID-19 increases so the ratio of true positives to negatives changes and the predictive value of having the disease, if you have no symptoms and test positive, increases.
But if you plan sensibly you can dramatically improve the value of screening the asymptomatic population at large.
If you have to test people who do not have symptoms then have a rapid recall system of the first screen positive individuals and re-test.
If you repeat the test on an asymptomatic individual and it is still positive then the positive predictive value increases dramatically. This is because the probability of being wrong twice is not simply an additive sum, the probability is much, much lower and follows Bayesian theory.
Based on current UK government figures on test accuracy and estimated prevalence rate on the 6th of October the probability of having the infection when tested as an asymptomatic is 7% if you retest on the same system and are positive the probability jumps to 39%.
Has anyone suggested this to Governments?
Ray Iles and Jonathan Iles
Definitions
Healthy: negative for disease
True positive (TP) = the number of cases correctly identified as infected
False positive (FP) = the number of cases incorrectly identified as infected
True negative (TN) = the number of cases correctly identified as healthy
False negative (FN) = the number of cases incorrectly identified as healthy
Sensitivity: The sensitivity of a test is its ability to determine the infected cases correctly. To estimate it, we calculate the proportion of true positive in patient cases. Mathematically, this can be stated as:
Sensitivity=TP/TP+FN
Specificity: The specificity of a test is its ability to determine the healthy cases correctly. To estimate it, we calculate the proportion of true negative in healthy cases. Mathematically, this can be stated as:
Specificity=TN/TN+FP
Accuracy: The accuracy of a test is its ability to differentiate the infected and healthy cases correctly. To estimate the accuracy of a test, we calculate the proportion of true positive and true negative in all evaluated cases. Mathematically, this can be stated as:
Accuracy=TP+TN/TP+TN+FP+FN
Photo: Karolina Grabowska
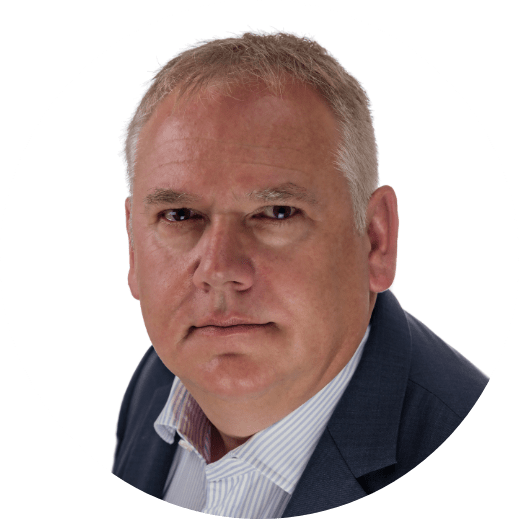
Professor Ray Iles
Head of Biomedical Science at NISAD
BSc in Bioanalysis, MSc in Immunology and PhD in Molecular Pathology